September 4, 2018
Mind-machine partnership
Past CBC Scholar, Adam Pah, NU, discusses potential benefits of collaborating with artificial intelligence
In a fascinating interview conducted by Clare Milliken for Northwestern Now, two NU’s Kellogg School of Management professors, Brian Uzzi and Adam Pah, discuss the meaning of machine learning and artificial intelligence as seen today and as hoped to be developed in a not-too-distant future. The benefits of pairing humans with learning-capable machines are also addressed, especially in reducing human innate capacity for bias and in providing real time feedback, allowing for real time correction and optimization of the tasks on hand. Adam Pah received a CBC Scholar Award (2011-2012) to help support his PhD studies. The CBC is proud to have supported Adam in the past and congratulates him on the remarkable progress in his career!
The bright future of machine learning
Innovation experts discuss the wide-ranging benefits of pairing minds with machines
Northwestern Now | by Clare Milliken | August 20, 2018
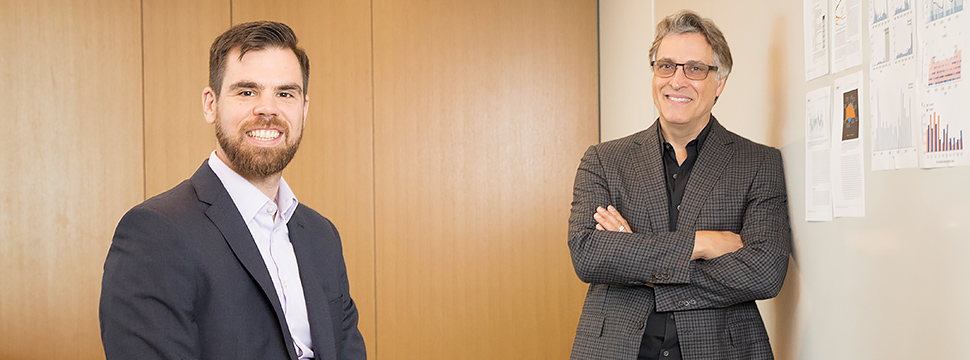
Brian Uzzi (right) is the Richard L. Thomas Professor of Leadership and Organizational Change at the Kellogg School of Management, NU. He is also the co-director of the Northwestern Institute on Complex Systems (NICO) and director of the Kellogg Architectures of Collaboration Initiative (KACI).
Adam Pah is a professor of management and organizations at the Kellogg School of Management. He is also the associate director of the Northwestern Institute on Complex Systems (NICO).
A decade ago, just three of the 2,500 students enrolled in leadership and organizational change professor Brian Uzzi’s classes knew about machine learning. Five years ago, three became 10%, and now, this topic is “the number one thing all students want to learn about,” Uzzi says.
Machine learning is definitely a hot topic these days, and both Uzzi and management and organizations professor Adam Pah (above left), who together have been studying machine learning for more than two decades, have some ideas about its benefits, pitfalls and vast potential.
How do you define machine learning? How do you define artificial intelligence (AI)?
Pah: In the common sphere, the terms “machine learning” and “artificial intelligence” are used pretty interchangeably. I think of artificial intelligence as a nonhuman entity performing a task with what would appear to be the intelligence of a human. Really, it all depends on who you ask — but in my classes I use the term “machine learning,” which I define simply as “an algorithm that learns from empirical data.”
Why should we care about machine learning?
Uzzi: When you look at machine learning, it is like a giant tsunami wave — you’ve got universities investing hugely in it, across engineering, computer science, the social sciences. Then you’ve got the government, which is making giant investments in artificial intelligence and machine learning. And then you’ve got private corporations — Google, Amazon, Facebook, many companies in China. And with all of that investment, chances are things are going to move at a tremendous rate.
Pah: Machine learning is more and more pervasive and seeping into everyone’s lives. Banks use machine learning to determine your credit limit. Facebook uses it to filter content. Machine learning is already deployed across all sorts of platforms, and it’s going to affect all of us eventually. From a business perspective, machine learning is already too large to ignore.
Can widespread use of machine learning be a bad thing?
Pah: Machine learning is a tool, and I think widespread use of any tool could be a bad thing, depending on how we choose to use it. For the most part, machines and machine learning can really only be as good as we are. They’re not all bad, but they’re not all great either. Machines aren’t solving hunger on their own tomorrow, but we can use them to help us do things.
Uzzi: I think the technology is also going to allow us to look at ourselves in a new way. These machines have the potential to show us when we’re biased, and we’ve never had that kind of feedback at our disposal before.
Let’s say you’re a judge, and you’re wearing some kind of biosensor that can detect blood pressure spikes when you’re confronted with a person of a particular ethnicity or gender. This machine could show you that you have a subconscious reaction that suggests you perceive a certain group as threatening. You’d never recognize that on your own. So, there’s feedback that the machines can give us.
Where do you see machine learning headed next?
Uzzi: One of the things I’m most excited about is what I call the mind+machine partnership. In the future, I believe one of the most important people in your team — one of the most important people in your network — is not going to be a person at all. It’s going to be a machine, and that machine is going to be highly customized to the way your mind processes information, its biases, its incomplete knowledge, its frailties. That machine is going to help you overcome those problems in the way other people have done in the past. And when we can all have this boost in our ability to absorb more knowledge and make sense of it, I think we will really have a whole new world of collaboration.
Can you give us an example?
Uzzi: I think the first place we’re going to see this synergy between humans and machines is in health care. I think we’ll have sensors on our bodies that collect biodata: blood pressure, cholesterol, inflammation, platelet counts, et cetera. We’ll have all that information constantly being collected on us, and neither you nor your doctor will be able to analyze it all. You will need your own artificial intelligence partner to make sense out of it and show you patterns. You’ll share all this data with your doctor before your annual visit, and your doctor will use his AI machine to figure out what’s going on.
This has the potential to fundamentally change medicine. Think about it: You see a doctor once a year, and you try to summarize 365 days in five minutes. Your sampling is probably not very good; you probably recall more recent events and issues and overlook others. But when your doctor has access to a stream of data, collected over the year, that’s when they can really see what’s happening with you.
But there’s more to mind+machine partnerships than just biodata, right?
Pah: Definitely. Mind+machine partnerships are useful in any situation where you’re dealing with a large amount of data, constrained time, or the need for continuous coaching or training.
Using machine partners can help us make better choices, decisions and products, with a key advantage being that machines can work on demand, while another human might not be able to learn or respond as quickly.
An example I use in class is the use of mind+machine to improve food safety at restaurants. Computers use pre-existing historical data to predict when to inspect restaurants. The computer can identify a restaurant that’s likely to have a violation, and then human inspectors can follow up. And this partnership has done a much better job — inspectors were getting to restaurants that had critical violations about a week earlier than they would have if they had just gone with the normally generated schedule.
Uzzi: Mind+machine partnerships will impact decision-making of all kinds — from health care to business to journalism to everyday life. These partnerships will help us overcome human biases and expand our awareness of things we previously ignored, overlooked or didn’t have the capacity to consider. These partnerships will allow us to scale up human effort and work bigger, smarter and more efficiently across all fields.
Source:
Adapted (with modifications) from the Northwestern Now, by Clare Milliken, published on August 20, 2018.
Featured scientist(s) with ties to cbc:
Adam Pah, NU
- CBC Scholar Award (2011-2012):
▸ CBC Scholar: Class of 2011
Adam Pah, Department of Chemical and Biological Engineering, NU; Advisor: Luis Amaral